
[ad_1]
Attributing the quip to former British prime minister Benjamin Disraeli, Mark Twain as soon as wrote, “There are three sorts of lies: lies, damned lies, and statistics.” Given the foundational significance of statistics in fashionable science, this quote paints a bleak image of the scientific endeavor. Fortunately, a number of generations of scientific progress have proved Twain’s sentiment to be an exaggeration. Nonetheless, we shouldn’t discard the knowledge in his phrases. Whereas statistics is a vital instrument for understanding the world, using it responsibly and avoiding its pitfalls requires a fragile dance.
One maxim that must be etched into the partitions of all scientific establishments is to visualize your knowledge. Statistics focuses on making use of goal quantitative measures to grasp knowledge, however there isn’t any substitute for really graphing it out and getting a have a look at its form and construction with one’s personal eyeballs. In 1973, statistician Francis Anscombe feared that others in his subject have been shedding sight of the worth of visualization, writing, “Few of us escape being indoctrinated” with the notion that “numerical calculations are precise, however graphs are tough.” To quash this fantasy, Anscombe devised an ingenious demonstration often called “Anscombe’s quartet.” Along with its wacky successor, the “datasaurus dozen,” nothing extra dramatically communicates the primacy of visualization in knowledge evaluation.
To understand Anscombe’s quartet, let’s slip into the lab coat of a scientist. Suppose you’re within the relationship between how a lot individuals train and the way a lot they sleep. You survey a random pattern of the inhabitants about their habits, report their solutions in a spreadsheet and run the outcomes by means of your favourite statistics software program. The ensuing abstract statistics appear like this (that is simply an instance and isn’t based mostly on actual knowledge):
Hours of train per week – Common: 7.5, Customary deviation: 2.03
Hours of sleep per day – Common: 9, Customary deviation: 3.32
Correlation between the 2: .816
On common, the individuals in your pattern train 7.5 hours per week and sleep 9 hours per day. Customary deviation measures how a lot variation there may be in your pattern. For each variables it’s reasonably sized, indicating that most individuals you surveyed don’t veer an excessive amount of from the averages. The 2 are extremely correlated, which means that individuals who train extra are additionally prone to sleep extra. The software program additionally outputs a line of finest match, which describes the overall development of your knowledge as the road under:
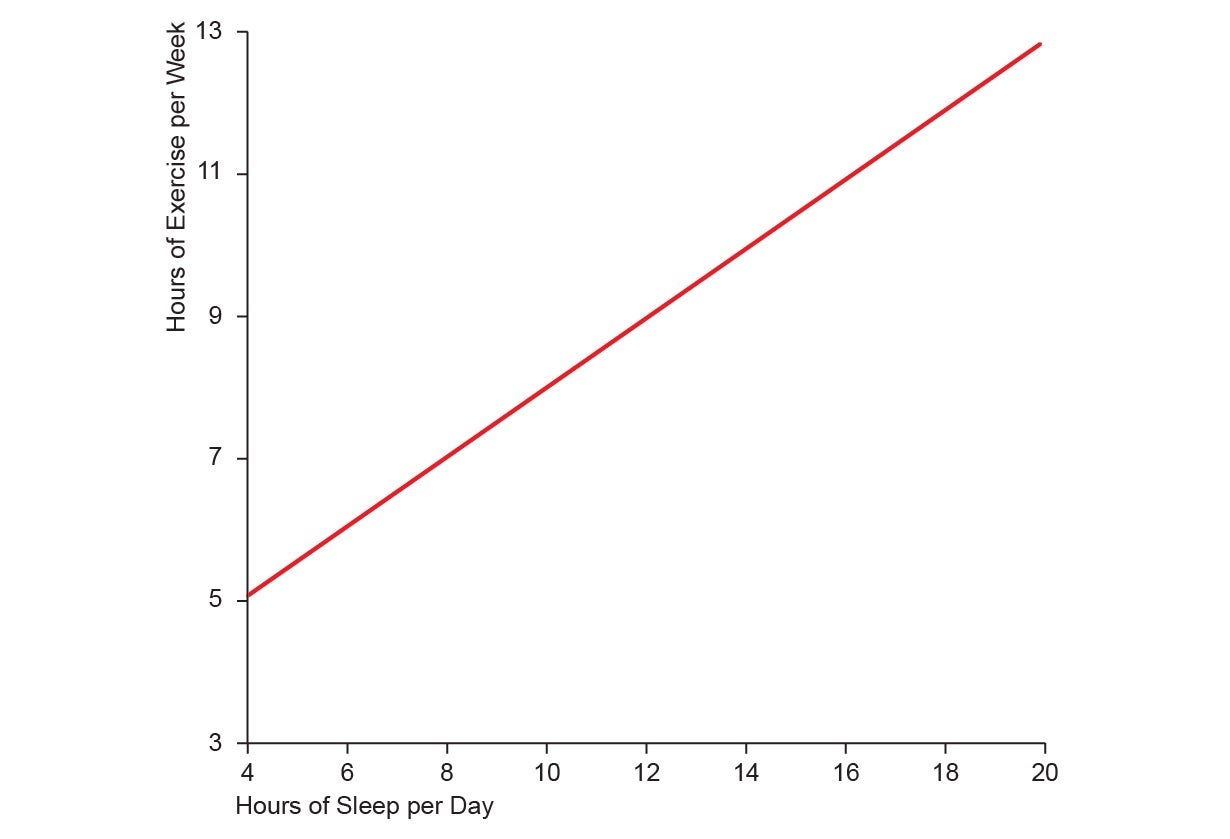
Given this abstract, it could be tempting to suppose that the info look one thing like this:
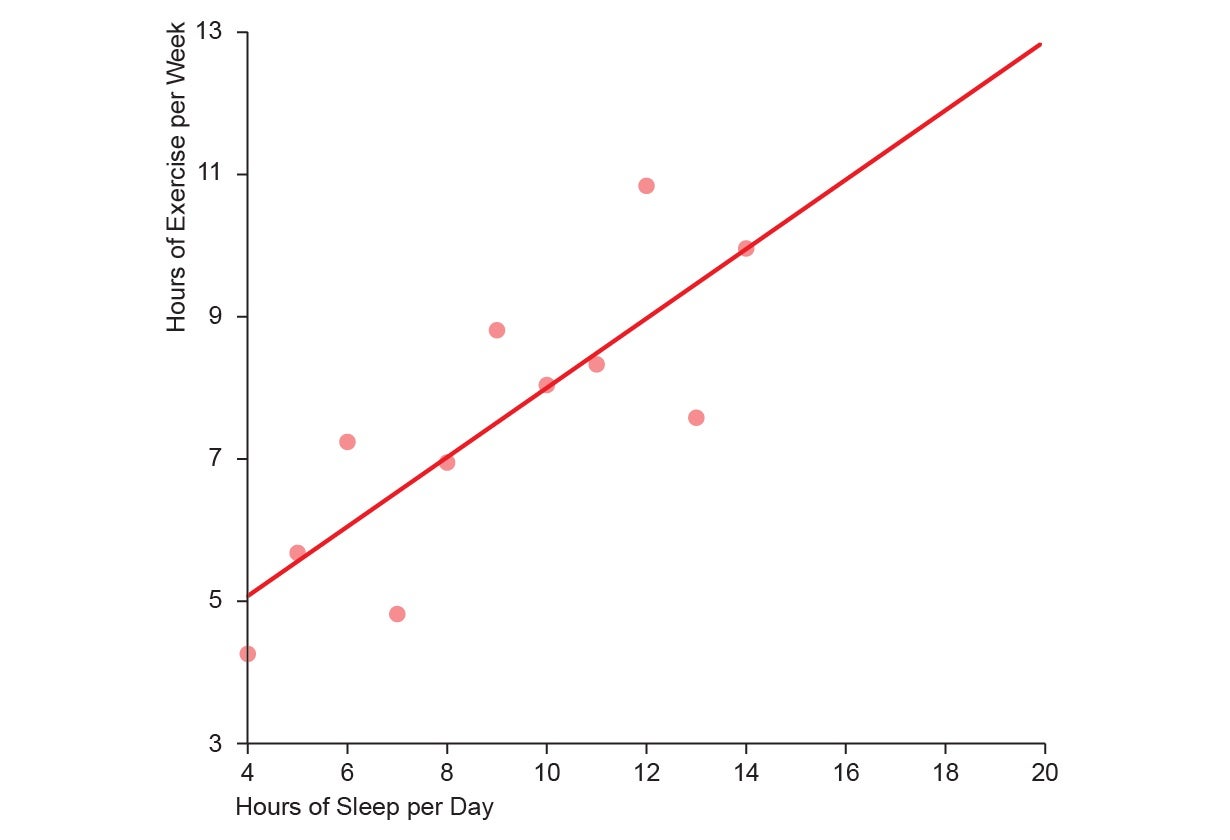
Every dot represents one individual in your survey and is positioned based on their private sleep and train habits. The above depicts a powerful upward linear development, suggesting that as individuals train extra, additionally they sleep extra (maybe as a result of each are indicative of usually wholesome life or as a result of exercises are fatiguing) with little random variations attribute of the messy actual world. Anscombe confirmed that, amazingly, all 4 datasets under have the an identical abstract statistics:
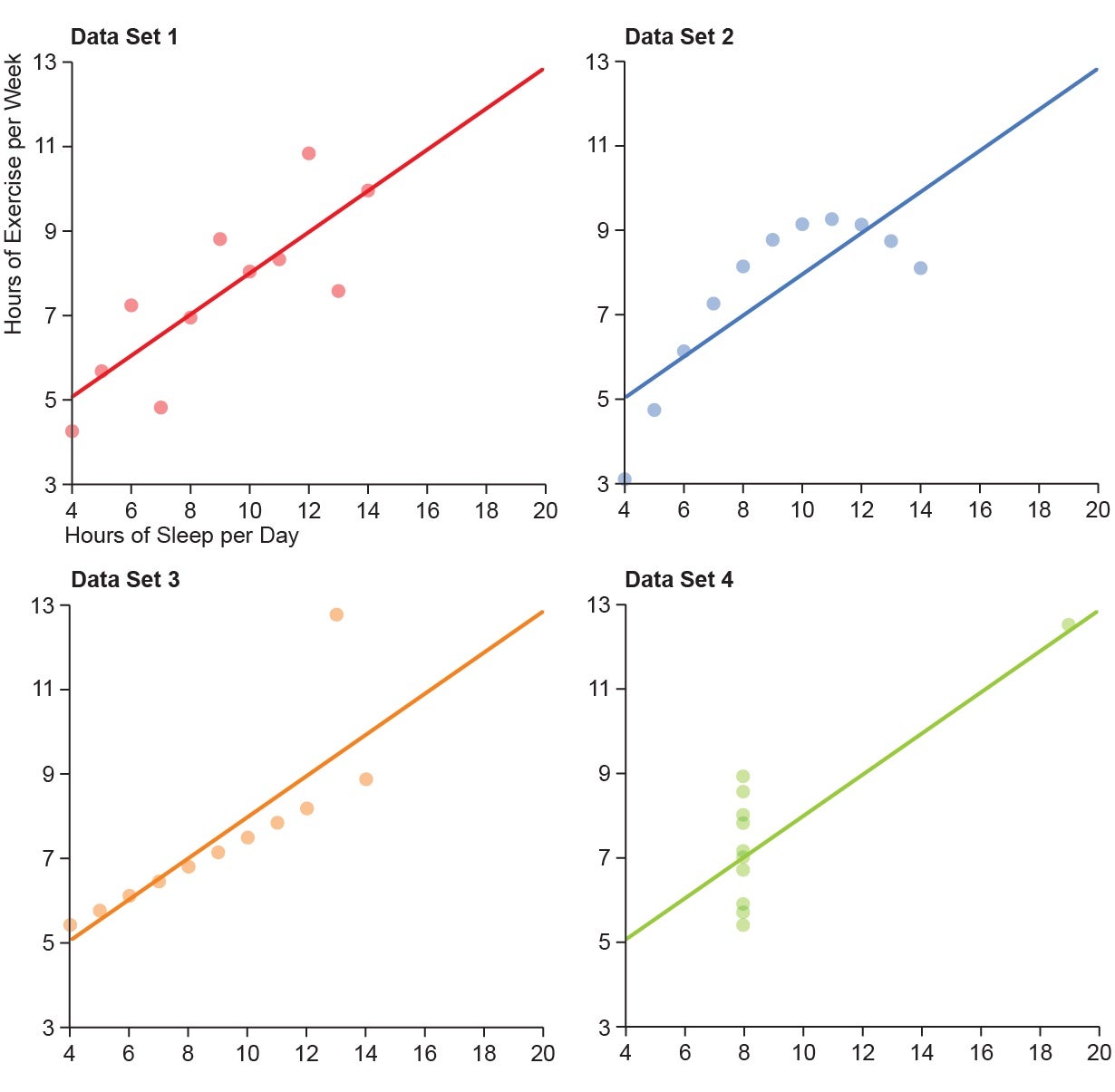
(Anscombe’s knowledge units don’t really correspond to any particular experiment, we’ve contrived one right here for illustrative functions). Dataset two, regardless of having the identical statistical profile as dataset one, tells a very completely different story when plotted. Right here, the connection is clearly not linear and for some motive train begins to taper off for individuals who sleep essentially the most (maybe as a result of sleeping a lot leaves little time for different actions). Plot three exhibits an ideal linear relationship, with one outlier who workouts an irregular quantity and skews the outcomes. Plot 4 exhibits that nearly all people sleeps precisely 8 hours per day and it has no relationship to how a lot they train, whereas one individual within the pattern sleeps all day and presumably spends all of their waking time exercising. Discover how we really draw very completely different conclusions from the identical statistics as soon as we visualize the info.
Regardless of its reputation, no one is aware of how Anscombe concocted his well-known quartet. Justin Matejka and George Fitzmaurice sought to rectify this and took the idea to its excessive, demonstrating a normal function methodology for taking any knowledge set and reworking it into any goal form of your selecting whereas preserving whichever abstract statistics you need (as much as two decimal locations). The outcomes are the “datasaurus dozen.”
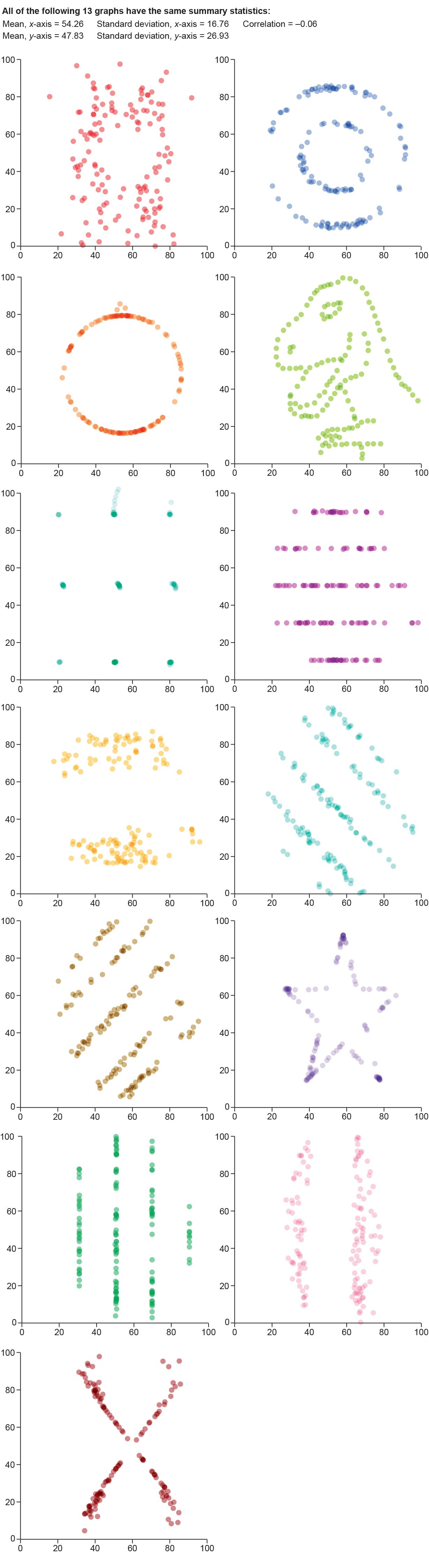
The entire scatter plots above have the identical abstract statistics! Astute readers may discover that it’s really a datasaurus baker’s dozen. The dinosaur dataset was really the seed from which all the others have been generated (it’s an homage to knowledge visualization skilled Alberto Cairo’s tongue-in-cheek T. rex dataset.) This great gif exhibits the plots reworking into one an one other and monitoring the altering stats on the aspect. Even the transition frames protect the statistics. Clearly abstract statistics alone inform an insufficient story.
Anscombe would most likely be proud that his quartet lives on as a standard pedagogical demonstration in fashionable statistics courses. As Yogi Berra stated: “You may observe lots by watching.”
[ad_2]